A best-in-class data platform that provides an integrated view of all children and early childhood providers.
- Improve decision-making.
- Identify disparities in early childhood education access and needs.
- Enhance program performance.
- Optimize public policies.
- Enable multiple agencies/departments to work together more efficiently to improve child and family outcomes.
Features
A best-in-class data platform that provides an integrated view of all children and early childhood providers.
Cloud Efficiency
More cost effective, easier to deploy, and offers better tools than on-premises approaches.
Integrated Enterprise
Provides seamless enterprise-level access and integration across agencies.
Early Childhood Insights
Captures provider and child-level data to inform early childhood policy.
Longitudinal Tracking
Stores and maintains longitudinal data to track, understand, and make accurate forecasts on the early childhood market.
Adaptable Solution
Adaptable to reflect early childhood programs, eligibility criteria, and policies in user’s state, region, or city.
Scalable Solution
Scales flexibly with your organization.
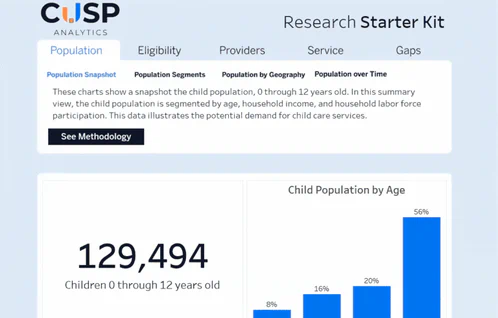
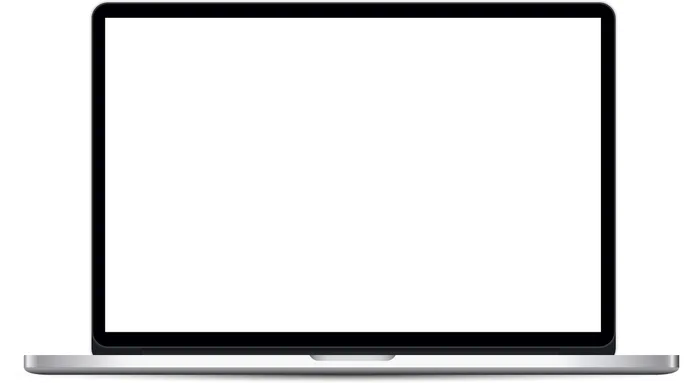
The Research Starter Kit allows users to analyze CUSP data on the child population, provider landscape, and funding programs.
- Intuitive interface
- Powerful analytical options
- Ability to study trends over time
- Regularly refreshed data
The CUSP Analytics Library is a unified interface where users search, build, and store analytics and visualizations.
3Si’s standard analytical package includes:
- Comprehensive views of early childhood services
- Funding programs (e.g., federal and state child care subsidies)
- Access deserts, and gaps in availability of affordable early childhood services, particularly in socio-economically vulnerable areas.